ADMM for High-Dimensional Sparse Penalized Quantile Regression
Two variants of the ADMM algorithm for solving the penalized quantile regression
By Yuwen Gu, Jun Fan, Lingchen Kong, Shiqian Ma, and Hui Zou in Research Quantile regression
May 9, 2018
Gu, Y., Fan, J., Kong, L., Ma, S., & Zou, H. (2018). ADMM for high-dimensional sparse penalized quantile regression. Technometrics, 60(3), 319–331.
Abstract
Sparse penalized quantile regression is a useful tool for variable selection, robust estimation and heteroscedasticity detection in high-dimensional data analysis. The computational issue of the sparse penalized quantile regression has not yet been fully resolved in the literature, due to non-smoothness of the quantile regression loss function. We introduce fast alternating direction method of multipliers (ADMM) algorithms for computing the sparse penalized quantile regression. The convergence properties of the proposed algorithms are established. Numerical examples demonstrate the competitive performance of our algorithm: it significantly outperforms several other fast solvers for high-dimensional penalized quantile regression. Supplementary materials for this article are available online.
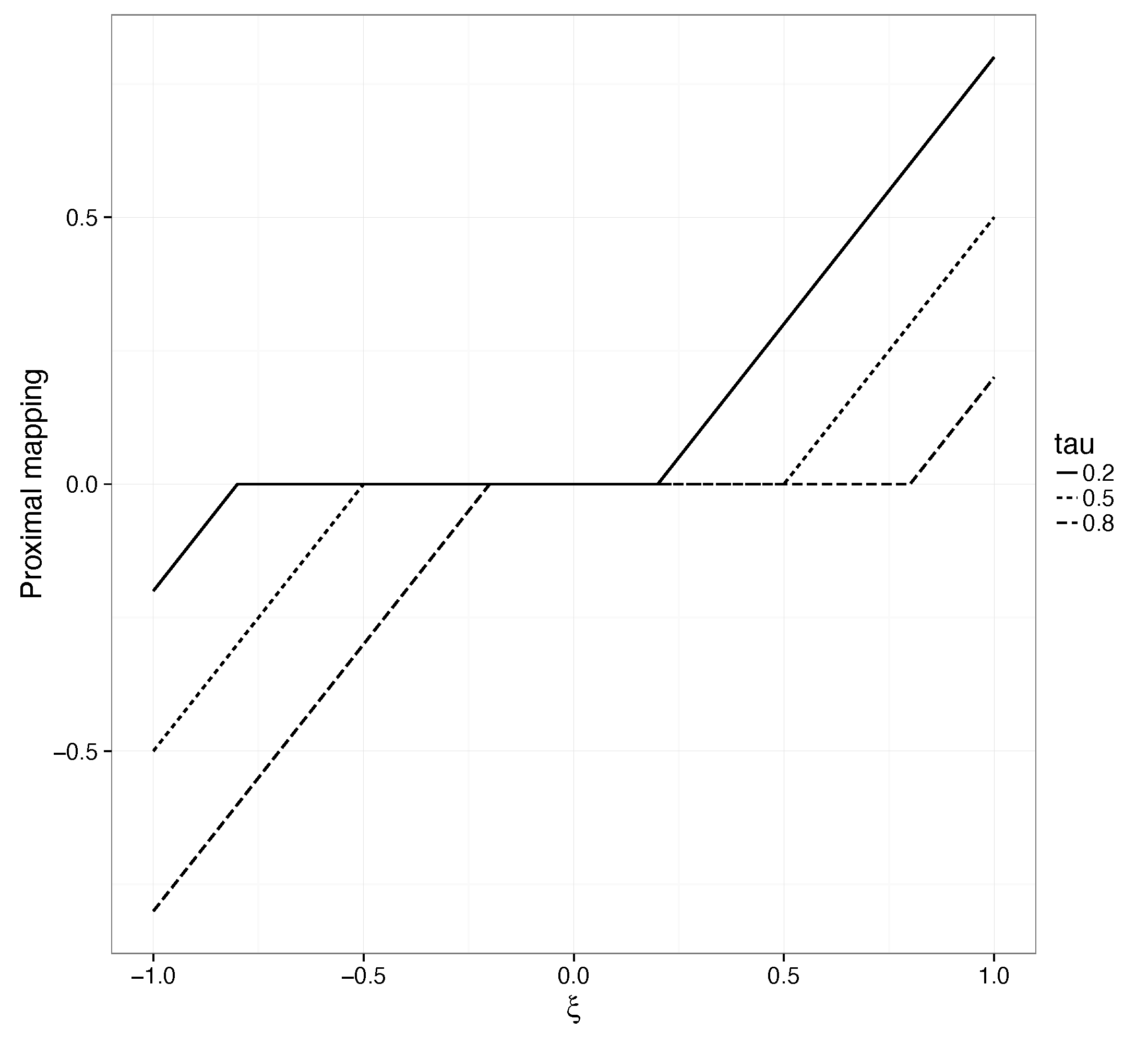